A Guide to AI Terminology for Businesses
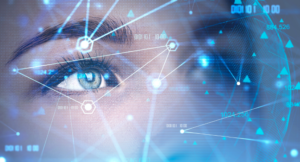
As artificial intelligence (AI) continues to shape the tech landscape, the terminology around it can be challenging to navigate. Businesses now face a surge of AI tools and platforms designed to enhance productivity, streamline tasks, and provide innovative solutions. This guide covers key AI terminology for businesses to help you understand what’s happening in this dynamic field.
What Exactly is AI?
AI, or artificial intelligence, is a field within computer science focused on creating systems that simulate human intelligence. The technology can range from simple task automation to complex data analysis and decision-making tools that improve business processes. Here’s a look at foundational AI terminology for businesses:
Artificial General Intelligence (AGI): A theoretical form of AI capable of performing any intellectual task a human can. While still speculative, AGI represents the ultimate goal for many researchers.
Bias in AI: The presence of prejudice in AI outputs caused by skewed training data or flawed algorithms. Understanding and mitigating bias is crucial to ensuring fairness in AI applications.
Deep Learning: A subset of machine learning that uses artificial neural networks to analyze vast amounts of data. Deep learning powers many AI innovations, from image recognition to speech processing.
Generative AI: AI that creates new content, such as text, images, and videos, based on training data. Examples include tools like ChatGPT and image generators like DALL-E.
Hallucinations in AI: The tendency of generative AI models to confidently provide incorrect or nonsensical answers due to gaps in their training data or overgeneralization.
Machine Learning (ML): A method of training algorithms to recognize patterns in data and improve performance over time. ML underpins many AI technologies, from spam filters to recommendation systems.
Natural Language Processing (NLP): The ability of AI to understand, interpret, and respond to human language. NLP drives applications like virtual assistants, chatbots, and speech-to-text software.
Retrieval-Augmented Generation (RAG): An approach where AI models retrieve external data in real time to enhance their accuracy and provide contextually relevant responses.
Understanding AI Models
AI models are core to how AI systems work. Here are some key types of models and their purposes:
Diffusion Models: These models generate media content, such as images, from text prompts by progressively improving from noise. They’re widely used for graphic and video generation.
Foundation Models: Trained on massive datasets, foundation models serve as the base for various AI applications, with examples like OpenAI’s GPT and Google Gemini. Foundation models enable versatility, making it easier to adapt AI tools to different tasks.
Frontier Models: A term for cutting-edge AI models under development, these represent the future of AI capabilities. While not yet widely available, they are designed to push the boundaries of current technology and solve increasingly complex problems.
Generative Adversarial Networks (GANs): GANs create realistic images, videos, or audio by having two neural networks compete against each other. They’re widely used in digital content creation, marketing, and media enhancement.
Knowledge Graphs: These systems organize and link information, offering contextual insights. Used in search engines, business intelligence, and customer support, they improve data navigation and accessibility.
Large Language Models (LLMs): LLMs process and generate human-like text. They power tools like ChatGPT, Bard, and Gemini, making them essential for natural language processing, customer support, and content generation.
Recommendation Systems: Designed to predict user preferences, these models personalize experiences on platforms like Amazon, Netflix, and Spotify. Businesses use them to improve customer engagement and retention.
Reinforcement Learning Models (RL): These models learn through trial and error by interacting with their environment, making them ideal for decision-making tasks in gaming, robotics, and logistics optimization.
Transformer Models: The architectural foundation for most AI models, transformers excel at sequence-to-sequence tasks like text translation, summarization, and question answering.
The Training Process
AI models undergo a training process where they learn from data to make accurate predictions and generate relevant responses. This involves analyzing vast datasets to identify patterns and relationships. Here’s a closer look:
- Pretraining:
- Models are exposed to large datasets (e.g., text, images, or audio) to build a general understanding of information.
- This step creates a broad foundation for the AI’s capabilities.
- Fine-tuning:
- After pretraining, models are customized for specific tasks, such as analyzing customer trends or automating responses.
- Fine-tuning helps align the AI’s outputs to the unique needs of a business.
- Parameters:
- Parameters are the internal variables that determine how AI processes data.
- Businesses often prioritize high-quality training data and careful parameter optimization over sheer parameter count to improve accuracy.
- Data Quality and Ethics:
- High-quality and representative training data are essential to minimize errors and biases.
- Businesses must also comply with data privacy regulations (e.g., GDPR, CCPA) when using sensitive information.
- Resources:
- Training AI models requires substantial computing power, often supported by cloud services like AWS, Google Cloud, or Microsoft Azure.
- Cloud solutions make it easier and more cost-effective for businesses to scale AI initiatives.
By understanding and investing in these elements, businesses can train AI models to improve efficiency, automate processes, and uncover meaningful insights.
Commonly Used AI Applications in the Business World
Many companies offer AI-powered tools that transform daily business tasks. Here are some of the major players:
- Amazon Web Services (AWS): Provides tools like SageMaker and Rekognition for cloud-based AI solutions.
- Anthropic / Claude: Focused on advanced conversational AI with backing from Amazon and Google.
- Apple / Apple Intelligence: Integrates AI into Apple’s ecosystem, improving Siri and other tools.
- Google / Bard: Google’s conversational AI platform, optimized for generating responses and assisting with research.
- Google / Gemini: Powers Google Workspace and various AI tools.
- IBM Watson: Offers advanced analytics and AI solutions for industries like healthcare and finance.
- Meta / Llama: Open-source AI model with applications in research and business experimentation.
- Microsoft / Copilot: Integrates AI across Microsoft 365 and Teams to enhance productivity.
- OpenAI / ChatGPT: Ubiquitous for natural language processing and content generation.
- Salesforce Einstein: CRM-focused AI offering predictive insights and automation.
- xAI / Grok: Elon Musk’s conversational AI model, aiming to rival established platforms like ChatGPT.
- Zoom AI Companion: Enhances virtual collaboration with meeting summaries and action items.
Incorporating these AI tools can help businesses improve efficiency, automate tasks, and better understand data patterns.
We hope you found our guide on AI terminology for businesses helpful! At GreenBean IT, we assist companies in leveraging AI for meaningful productivity gains. Contact us to learn more about integrating AI into your tech strategy.